Masoud Ghahremanloo’s Work Published in Journal of Geophysical Research: Atmospheres
A University of Houston doctoral student has developed a more accurate artificial intelligence (AI) method for reading amounts of ground-level nitrogen dioxide (NO2) with the help of satellite technology.
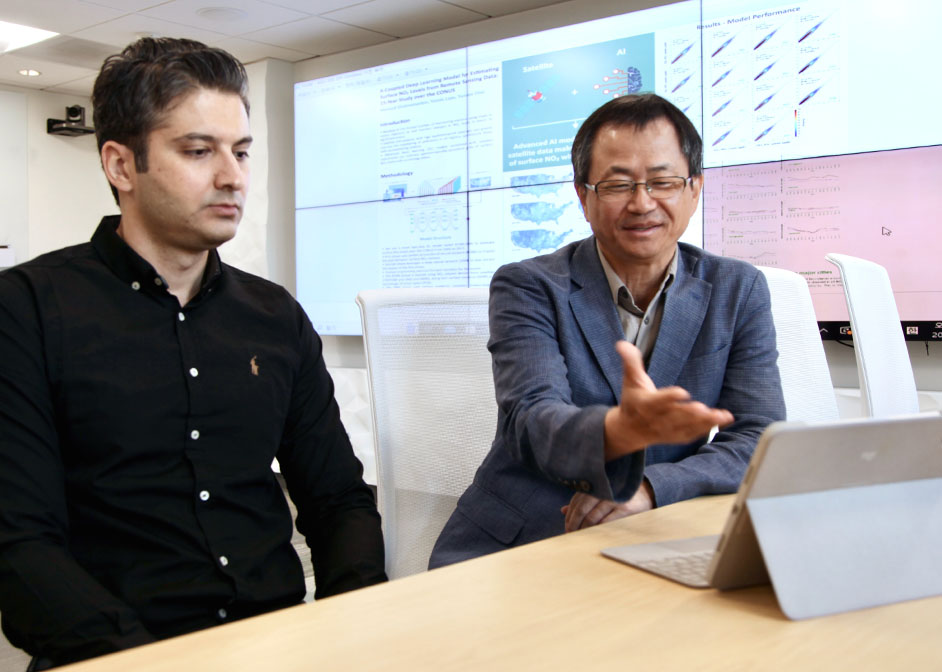
Masoud Ghahremanloo worked with associate professor Yunsoo Choi of the UH Department of Earth and Atmospheric Sciences to create a model designed to give more accurate estimates of NO2 concentrations on the Earth’s surface. Additional UH College of Natural Sciences and Mathematics doctoral students, Yannic Lops, Seyedali Mousavinezhad, and Jia Jung, also collaborated in the study.
Ghahremanloo’s work was published in the national scientific journal, Journal of Geophysical Research: Atmospheres.
Detecting NO2 Through Artificial Intelligence
NO2 is a pollutant formed from emissions at ground level from vehicles, power plants, industry and off-road equipment.
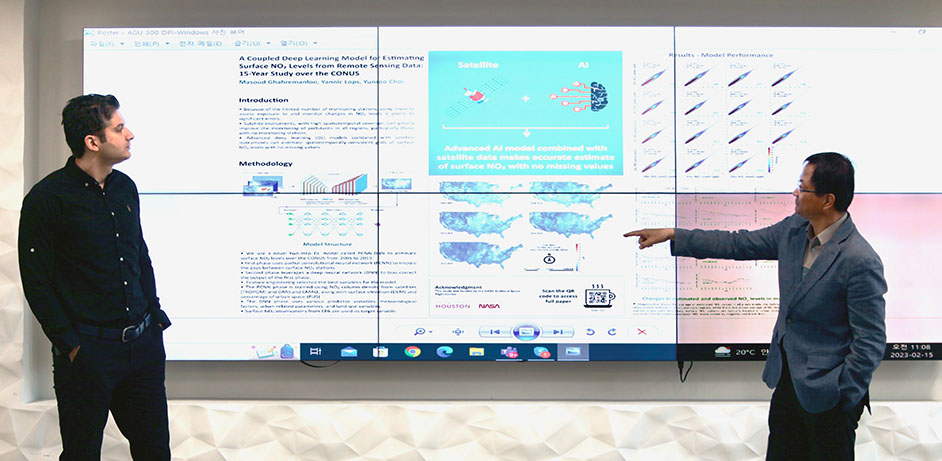
Before data was obtainable by satellites, gathering accurate measurements of NO2 in all regions was a challenge due to the sporadic locations of monitoring stations on the ground.
Ghahremanloo compares the behavior of the new model used in the study to that of an AI model to detect features of the human face.
“The example AI model, which fills the gaps inside pictures of the human face, is trained to know where the eyes, nose and mouth should be placed on a face,” said Ghahremanloo. “For NO2 detection, our two-step, deep learning model is trained on satellite data to capture the spatial pattern of NO2 concentrations in all regions, particularly those without surface monitoring stations.”
Filling the Data Gaps
In this model, the AI model learns from satellite data, whether a specific region is a densely populated or remote area.
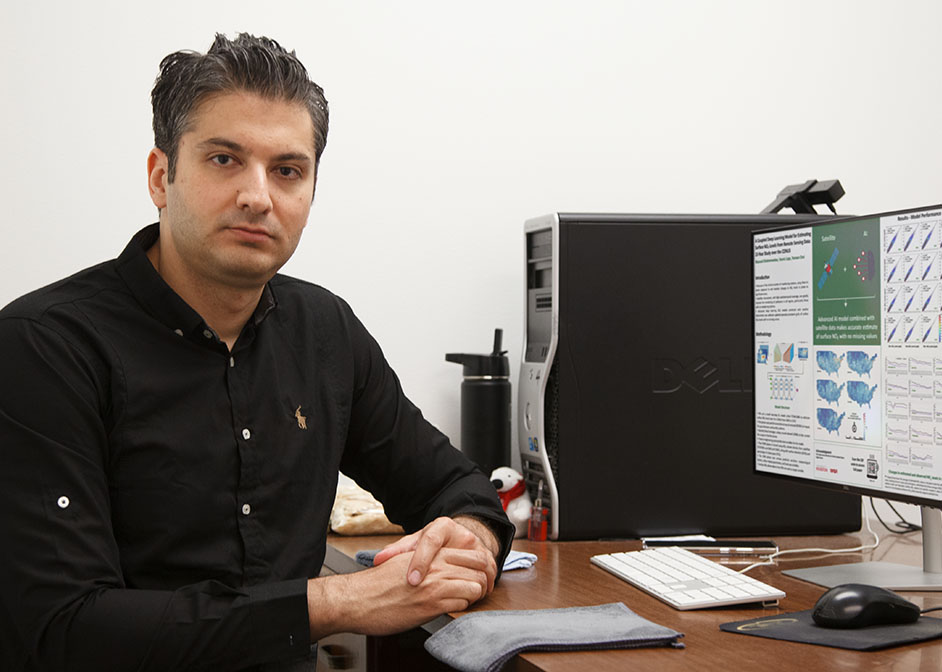
“Surface monitoring stations can’t be installed in all places to help with monitoring,” said Choi. “For this reason, satellites are the main tools used in this study.”
To help create the new model, the UH team focused solely on NO2 levels in the contiguous United States, using data compiled from 2005 until 2019 from ground monitors and satellites.
The study’s results have helped equip regional leaders with better knowledge that allows them to make more informed decisions when it comes to reducing NO2 concentrations for the sake of human health.
Pros and Cons of Satellite Data Collection
While the accuracy of satellite data is dependable, the collection of data hinges on the weather.
“If cloudy conditions exist, it can interfere with the process,” said Ghahremanloo.
Signals from satellites are unable to penetrate the clouds to obtain an accurate NO2 reading on the surface.
Future of Data Gathering
A new satellite set to launch in April could lessen the impact of weather as an obstacle to gathering data.
NASA’s TEMPO (Tropospheric Emissions: Monitoring of Pollution) satellite is the first Earth Venture Instrument mission that will measure pollution in North America at a high resolution. The satellite will enable measurements on an hourly basis.
“TEMPO has higher sensitivity and more advanced technology than satellites we’ve used for this study,” said Choi. “The hourly measurements will enable us to obtain more accurate NO2 surface readings.”
- Chris Guillory, College of Natural Sciences and Mathematics