Team to Use Inverse Modeling of Remote-Sensing Data from AI and Numerical CMAQ Models
The University of Houston’s Atmospheric Artificial Intelligence Team, led by Yunsoo Choi, was awarded a $464,374 grant from NASA. The project is titled “Inverse Modeling Using Aura Omi and AI Deep Learning Approach to Investigate NOX, HCHO, and Ozone Sensitivity for a Historical Period over the Continental United States.” The team also received a $34,782 grant from Anyang University, which is for optimizing the emissions of PM2.5 ingredients over South Korea.
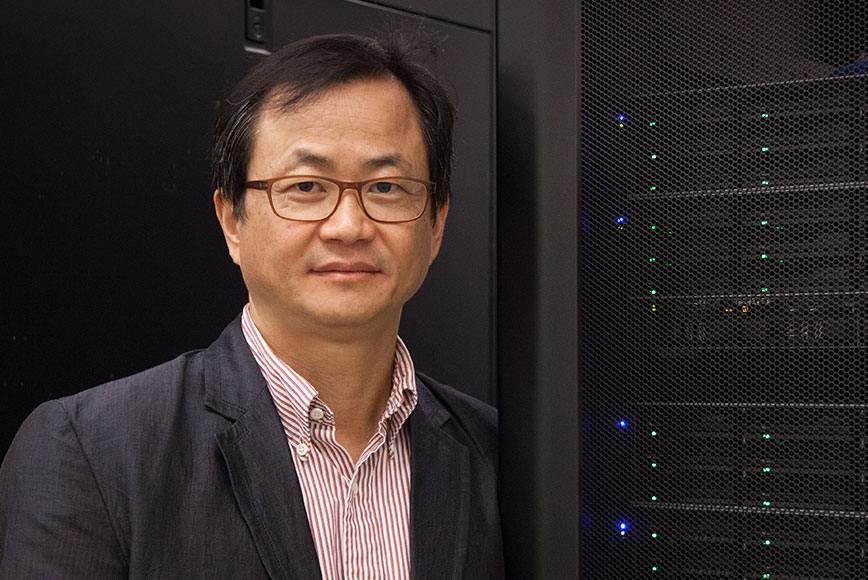
NASA Grant
The NASA grant expands research of Choi and his team in analyzing mobile and point-source emissions from anthropogenic and biogenic sources of ozone using remote sensing data with Artificial Intelligence (AI) and the Community Multiscale Air Quality (CMAQ) models. The project aims to expand the understanding of mechanisms and sensitivity of atmospheric constituent processes and to improve the numerical accuracy models of ozone and its ingredients for short-term and long-term modeling.
Obtaining Source Sensitivities Using CMAQ and AI models
The team aims to identify sources of nitrogen dioxide (NO2) and formaldehyde (HCHO) using the direct decoupled method (DDM). This method is used to estimate first-order sensitivities with respect to emissions and initial and boundary concentrations. This method will allow the team to more accurately obtain an optimized emission inventory for a region. To improve the processing time, the team will create AI-based DDM for the real-time and long-term estimation of emission inventory for the contiguous United States.
Anyang University Grant
This grant provides research funding for Choi and his team to analyze emission and precursors of PM2.5 using remote sensing data with the CMAQ and AI models. The project aims to expand the understanding of mechanisms and sensitivity of atmospheric constituent processes and improve the accuracy of numerical models of PM2.5 modeling.
Importance of PM2.5 Research
Particulate Matter (PM) at 2.5µm is created by secondary processes in the atmosphere through the emissions of precursors such as nitrogen oxides (NOX), sulfur dioxides (SO2), ammonia (NH3) and volatile organic compounds (VOCs).
PM concentrations have been linked to respiratory and cardiovascular diseases that lead to increased hospitalizations and mortality. Improving the understanding and estimation of PM concentrations will allow better policymaking in effectively reducing emissions that lead to high PM2.5 concentrations.
“Modelling and forecasting PM2.5 has been challenging using current numerical models,” said Choi, associate professor of atmospheric chemistry, remote sensing, and machine learning in the Department of Earth & Atmospheric Sciences. “Preliminary tests have shown that our AI systems have provided some improvements in accurately forecasting PM concentrations. The integration of CMAQ and AI, with the help of the grant, will allow us to better understand the interactions of emission precursors to PM concentrations for effective policymaking in reducing PM health-related issues.”
Inverse Modeling and Sensitivity Analysis
When using inverse modeling, scientists take the known outcome of a process (e.g., ozone concentration, PM2.5 concentration) and then reverse the atmospheric chemical processes to find the initial state (i.e., emissions such as VOCs, NOX, SO2, NH3) that led to the known atmospheric composition. These processes will be performed by the air quality model and expanded using AI for faster processing. Both systems will also allow the uncertainty analysis of emissions to be analyzed.
The Role of AI in Atmospheric Science
The project team has applied AI in atmospheric sciences for several years, with several papers already published showing improvements over conventional modeling methods in the short- and long-term forecasting of air quality and meteorology.
“While AI has shown great advancements and performance in our research, we believe the next step will be the integration of AI with numerical models (such as CMAQ),” said Choi. “The NASA and Anyang grants will allow us to develop a synergetic system of numerical and AI models by combining the advantages and alleviating the disadvantages of both models.”